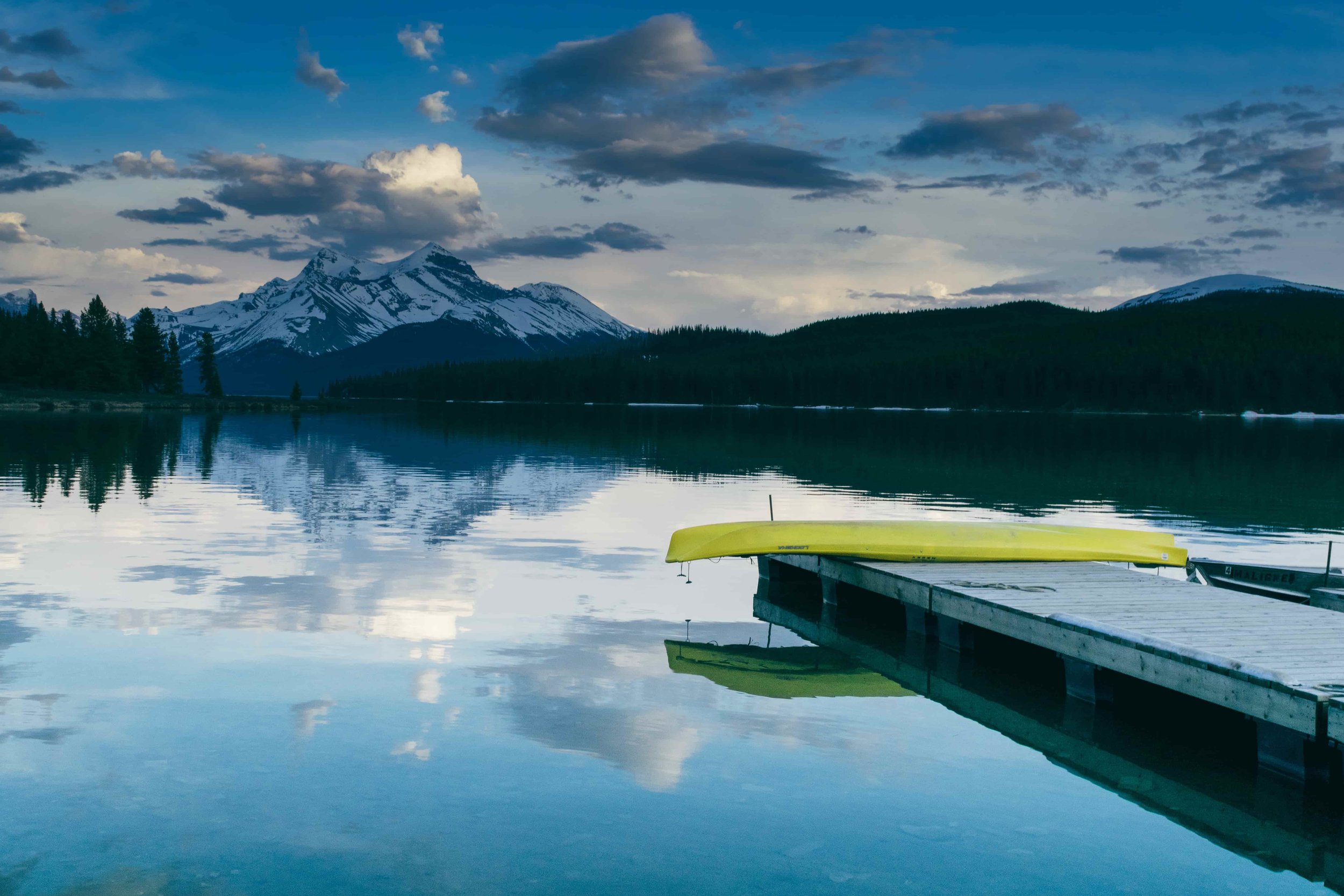
THE SUPPLY CHAIN BLOG
Interview: Why Does Supply Chain Analytics Really Matter?
Hakan Andersson discusses why Supply Chain Analytics really matters.
In the following video Hakan Andersson discusses why Supply Chain Analytics really matters.
Key Takeaways:
The Challenges of Making Sense of Big Data
Four Difficulties with Supply chain Data Analysis
Types of Supply Chain Data Analysis That Need to be Done
Why is it difficult to make sense of big data?
I would first like to say that it’s great to have big data. Now we have data, data on the deep level, we have the computer power to process the data. This means that we can actually, close to when things are occurring, establish what is reality. We don’t have to rely on established truths that, very often, turn out not to be truths, but rather to be company myths.
The risk here is that, with all this beautiful data available, it’s very easy to get carried away. There is also a risk that the data is available to a lot of people. Everyone who has the availability can draw their own conclusions. That is why the data needs to be curated; it needs to be handled by someone who has the expertise and the knowledge about the operations. Otherwise, the conclusions that anyone with access to the data are drawing might very well be wrong, and you’re comparing apples to pineapples.
We know from Establish-Davis Database where we’ve been following up logistics costs and services for almost forty years, that you’ve really got to know what is comparable and what is not.
It’s not fair to compare the logistics cost for a high-value, low-weight item like pharmaceuticals to an industry like the steel industry, where you have low value and high weight on your items. That makes a lot of difference, and it can give you a problem if you’re on the supply chain side of the company, because then you will be on the defense all the time, trying to explain why things are right or wrong, and you’ve got to be in command of what is important and what is right.
Difficulties with Supply Chain Data Analysis
A difficulty with sorting through the data is that, first, the data is never—I will say almost never—clean. Just the fact that you have a lot of data and it looks like it’s very accurate and valid doesn’t mean that it is, so you need to know and identify the weaknesses of the data, where the master files have been updated and where they haven’t and where you just have a place holder and where it’s real stuff.
Another difficulty with clean data is that even if the data is right, it might include extremes and it might include outliers that are distorting the results of the analysis you’re making. That makes that a first step to do to clean the data, identify the extremes, and know the weaknesses where you can use the data, where it’s not valid.
You have to decide what is important. Here, it’s easy to get lost in a lot of analysis, and you’ve got to know what is really making sense and where you get some output from to the analysis.
You must make the data comparable. For instance, you’ve got to make it comparable over time so that you know when the business has changed, and you’ve got to make adjustments to the trends. Trend analysis is the best way of monitoring and identifying where your measures to improve efficiency, for instance, are paying off and where you need to take a closer look.
Also, with big data, you want to benchmark between your entities and benchmark externally. Then you’ve got to make the data comparable between the entities.
An obvious and perhaps small example is when it comes to facilities. There is a cost to house the inventories in inventory where you might own some of the facilities yourself and you might rent some of the facilities. The actual cost that you should use might be the same, but the owned facility can come out very favorably in these comparisons. Here, you might want to create a fictional rent to level the playing field.
What Types of Supply Chain Data Analysis Needs to be Done and Why?
This of course, depends on the needs. What stage are you in? Are you turning around a company? Are you just keeping an eye on a well-functioning company? Have you had any mergers and so on?
The first area you want to look at is the cost and efficiency area. We tend to start out by looking at the logistics cost and structure per item, per customer, and per supplier, and we look at the order structure. What we try to look at is what is driving cost. By applying some rules of thumb or detailed knowledge that we have from other analyses, we can identify which items are profitable, which customers should we focus on, what suppliers do we have agreements with that makes the landed cost higher than we thought it would be.
If we know this, we can then make strategies for how we handle customers. It might be, which is often the case, that we have an order structure where we have a lot of small orders. The handling costs could be punitive for each order, so the small orders make the whole operation unprofitable. Knowing that, then we can find different ways to automate order handling or have free freight limits or have a handling charge.
There are a lot of ways that you can use this knowledge. If you look at the suppliers, very often, you find that it’s getting a bit unfocused, pointing in all directions, you have a lot of small suppliers. And it might make sense to have someone that is a bit more expensive, where the logistics are very efficient and very favorable for you.
When we look at efficiency, we, of course, focus on the handling cost and the facility cost, we’re looking at picking, receiving, administration. In this case we want to do trend analyses; we want to do benchmark internally between different entities and different facilities; we want to look at if there is any geographical area or country that is more favorable and more efficient.
When we get this in order, it’s very interesting to make an external benchmark. Where are your competitors? I mentioned earlier on the Establish-Davis Database, which is free of charge so you can get a good handle on how do you match up to your peers.
A specific area is the transportation cost. This is a cost item that, in most companies, are mostly external, which means that it’s money down on the bottom line. By analyzing the shipments and the needs for modes, you can find that you can often use cheaper modes, you can find potential consolidation areas, which we know, save a lot of money and, again, is on the bottom line.
There are trending analyses that are possible now that weren’t possible a number of years ago with, say, with the transportation. It used to be that it was impossible to really make a detailed analysis of how the actual freight billing compared to the agreements but now it’s possible.
What we know is that on average a company gets over-billed by 2 to 4 percent when you compare the actual shipment to the agreement and to constantly monitor this and follow up could be something that you outsource, but it’s also readily available in logistics control tower transportation-management systems, or you can, in some cases, do it yourself without those analysis tools.
If you’re looking at a more strategic level, we were touching on the analysis when it comes to suppliers, order structure, customers, items, assortment analysis, but a very lucrative area to look at is the distribution-network analysis. What you do is look at the…do I store the right amount of each item to reach a certain service level? Do I store the items where it’s most favorable cost-wise? Do I store them where it’s easiest and cheapest to ship to customers? All of this is possible to do with data that is readily available.
Conclusion
To summarize, the supply chain analytics that are possible to do now offers a great opportunity for you as a supply chain manager to get in to the driving seat. This area is an area where it’s very quantifiable, and you can put numbers on it. If you have the data, you can be in charge, you can define what is important, and you can drive and monitor the efficiency, and you can also be a good customer to the suppliers that are helping you.
Thank you very much for having me, and I am looking forward to talking to you again.
Data Driven Supply Chain Analytics Tools Are Not Enough
Supply Chain Analytics practitioners can learn a lot from Customer Analytics. The two domains are based on similar business fundamentals. What we find is that data and data-driven decision tools are not enough. The biggest difficulties in supply chain or Customer Analytics lie in the cultural practices of organizations.
Supply Chain Analytics practitioners can learn a lot from Customer Analytics. The two domains are based on similar business fundamentals. What we find is that data and data-driven decision tools are not enough. The biggest difficulties in supply chain or Customer Analytics lie in the cultural practices of organizations.
The following article was written by Maz Iqbal who provided his valuable perspective from a Customer Analytics point of view.
On LinkedIn, Don Peppers is sharing his perspective on making better decisions with data. This got me thinking and I want to share with you what showed up for me. Why listen to my speaking? I do have a scientific background (BSc Applied Physics). I qualified as a chartered accountant and was involved in producing all kinds of reports for managers and saw what they did or did not do with them. More recently, I was the head of a data mining and predictive analytics practice. Let’s start.
Data and data driven decision-making tools are not enough
Yes, there is a data deluge, and this deluge is becoming down faster and faster. Big enough and fast enough to be given the catchy name Big Data. What is forgotten is the effort that it takes to get this data fit for the purpose of modeling. This is no easy-cheap task. Yet, it can be done if you throw enough resources at it.
Yes, there are all kinds of tools for finding patterns in this data. And in the hands of the right people (statistically trained-minded, business savvy) these tools can be used to turn data into valuable (actionable) insight.
This is not as easy as it sounds. Why? Because there is shortage of these statistically trained and minded people: amateurs will not do, experts are necessary to distinguish between gold and fools gold – given enough data you can find just about any pattern. It statistical savvy is not enough you have to couple it with business savvy. Nonetheless, let’s assume that we can overcome this constraint.
The real challenge in generating data driven decision-making in businesses is the cultural practices. We do not have the cultural practices that create the space for data driven decision-making to show up and flourish. A thinker much smarter-wiser than me has already shared his wisdom, I invite you to listen:
"On the whole, scientific methods are at least as important as any other research: for it is upon the insight into the method that the scientific spirit depends: and if these methods are lost, then all the results of science could not prevent a renewed triumph of superstition and nonsense. Clever people may learn as much as they wish of the results of science – still one will always notice in their conversation, and especially in their hypotheses, that they lack the scientific spirit; they do not have the distinctive mistrust of the aberrations of thought which through long training are deeply rooted in the soul of every scientific person. They are content to find any hypothesis at all concerning some matter; then they are all fire and for it and think that is enough …….. If something is unexplained, the grow hot over the first notion that comes into their heads and looks like an explanation…."
- Nietzsche (Human, All Too Human)
It occurs to me that the scientific method never took route in organizational life. Put aside the rationalist ideology and take a good look at what goes in business including how decisions are made. I say you will find that Nietzsche penetrating insight into the human condition as true today as when he spoke it. The practice of making decisions in every organization that I have ever come in contact with is not scientific: it does not follow the scientific method.
On the contrary, managers make decisions that are in alignment with their intuition, their prejudices, and their self-interest. It is so rare to come across a manager (and organization) that makes decisions using the scientific method that when this does occur I am stopped in my tracks. It is the same kind of unexpectedness as seeing a female streaker running across the football pitch in a league match.
What are the challenges in putting data driven decision-making practices into place in organizations?
Technologists have a gift. What gift? The gift of not understanding, deeply enough, the being of human beings. Lacking this understanding they can and do (confidently) stand up and preach the virtues-benefits of technology. If life were that simple.
Truth shows up as attractive to those of us who do not have to face the consequences of truth. Data driven decision-making sounds great for those of us selling (making a living and hoping to get rich) data driven tools and services.
The challenge of putting in place data driven decision-making practices is that it disturbs the status quo. When you disturb the status quo you go up against the powerful who benefit from that status quo. Remember Socrates:
"The very nature of what Socrates did made him a disruptive and subversive influence. He was teaching people to question everything, and he was exposing the ignorance of individuals in power and authority. He became much loved but also much hated …. In the end the authorities arrested him for …., and not believing in the gods of the city. He was tried and condemned to die…"
- Bryan Magee, Professor
Beware of being successful in putting in place a culture of data driven decision making!
With sufficient commitment and investment you can put in place a data driven decision making culture. Like the folks at Tesco did. And by making decisions through harnessing the data on your customers, your stores, your products, you can outdo all of your competitors, grow like crazy and make bumper profits. Again, again, and again. Then the day of reckoning comes – when you come face to face with the flaws of making decisions solely on the basis of data.
Tesco is not doing so great. It has not been doing so great for several years – including issuing its first ever profits alert in 2012. What is the latest situation?Tesco has reported a 23.5% drop in profits in the first half of this year. What has Tesco been doing to deal with the situation? This is what the article says:
Last year, Tesco announced it would be spending £1bn on improving its stores in the UK,investing in shop upgrades, product ranges, more staff, as well as its online offering.
There are a number of flaws on data driven decision making. For one data driven decision making assumes that the future will be a continuation of the past. Which is rather like saying all the swans that we have come across are white, so we should plan for white swans. And then, one day you find that the black swan shows up! The recession and the shift in consumer behavior that resulted from this recession was the black swan for Tesco.
Furthermore, I hazard a guess that in their adoration at the pulpit of data driven decision making the folks at Tesco forgot the dimensions that matter but were not fed into the data and the predictive models. What dimensions? Like the customer’s experience of shopping at Tesco stores: not enough staff, unhappy staff, stores looking more and more dated by the day, the quality of their products...
It looks like the folks at Tesco did not heed the sage words of one of my idols:
Not everything that counts can be counted, and not everything that can be counted counts.
– Einstein
This article on ‘Musings on Big Data, Customer Analytics, and Data Driven Business‘ was written by Maz Iqbal who is an expert committed to helping executives, teams and organizations to do well by creating superior value for customers and enriching the lives of all stakeholders. His website is http://thecustomerblog.co.uk/